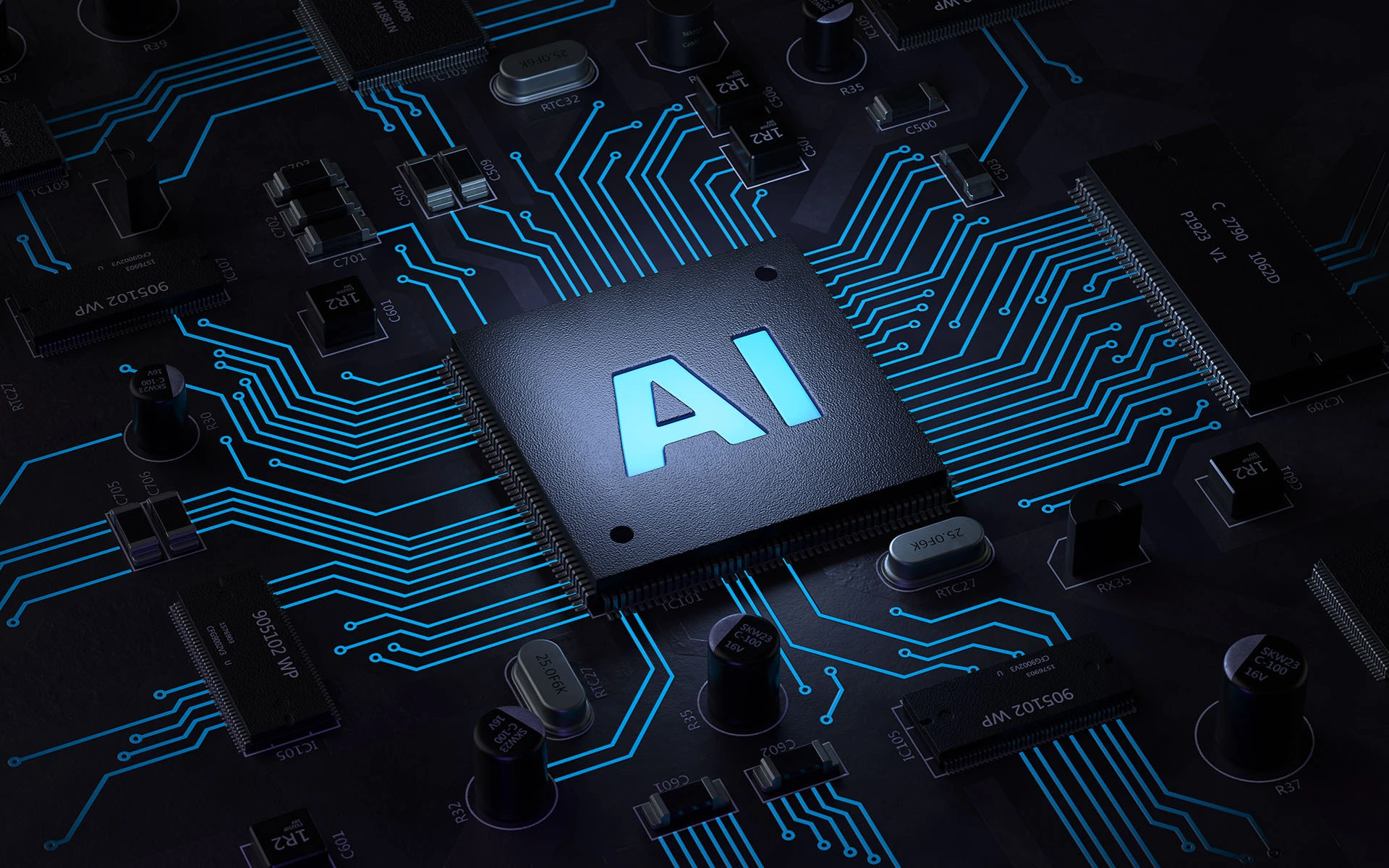
Artificial intelligence (AI) technologies are revolutionizing how electrical assets are maintained. AI enables organizations to gain a competitive edge by leveraging condition monitoring data to make better data-driven decisions, minimize downtime, optimize resources, and improve operational effectiveness.
Cloud computing, IoT, and broadband capacity have made these AI-enabled advantages possible.
Today, the world continues to evolve digitally due to the rise of remote working and the increasing spike in technological advancements such as robotics and artificial intelligence. The need for digitalization has become more significant across many industrial sectors, irrespective of the competitive landscape.
Digitalization is a prerequisite for every organization as they strive to become more efficient and achieve sustainability objectives. This evolution fosters the importance of improvement in thinking, structure, operation, and development by moving proactively towards more innovative ways of solving problems and adapting to the ever-developing digital landscape.
An increasing shift towards electrical power is inevitable for every organization, and to ensure optimal operation, reliability, and personnel safety, proper electrical infrastructure maintenance is as essential as maintaining a stable power supply.
Across many industries, organizations have relied on the traditional method of electrical asset maintenance. The various approaches to electrical asset maintenance typically involve scheduled, routine, and reactive ways of managing electrical equipment. Traditional asset maintenance revolves around periodic inspections, preventive maintenance, reactive repairs, or response to failure.
Over time, flaws have emerged with traditional asset maintenance approaches, which can result in unplanned downtime and higher maintenance costs. These flaws negatively impact the smooth-running operations for industries compared to modern, IIoT, and AI-driven maintenance approaches.
With the advent of Artificial Intelligence (AI), the landscape of critical asset maintenance has undergone a transformative shift, which is also evident in electrical assets. Electrical assets are now harnessing the predictive capabilities of sensors for better asset monitoring, providing alarm notifications and data-driven insights, resulting in the enhanced efficiency of electrical assets.